Every company has assets — financial investments, physical equipment, or intellectual property that need protecting and optimizing to contribute to your business’s success. But when portfolios slip, or equipment fails, the fallout can ripple across your operations before anyone has time to react.
To stay ahead of those disruptions, enterprise companies use artificial intelligence for a clearer, faster way to manage their assets. And it’s far from a passing trend — 73% of asset and wealth management firms say AI is the most transformational technology they’ll adopt in the next few years.
This guide explains AI in asset management in more detail, including how it works, its benefits, and the biggest challenges asset teams face in adopting the technology. We’ll also explore how platforms like monday service can support your enterprise asset management teams.
Try monday serviceWhat is AI in asset management?
AI in asset management allows business leaders to use intelligent systems to manage and optimize their investments. Instead of tracking asset status or performance manually, AI provides real-time visibility into your business data, enabling teams to act on what’s happening now — not last week.
Typically, AI can support organizations in managing a wide range of asset types, including:
- Financial assets: Portfolios, investments, securities, and other market-based holdings
- Operational assets: Equipment, machinery, tools, and other inventory resources used in daily operations
- Digital assets: Software licenses, data subscriptions, service level agreements, cloud services, and digital tools
- Physical infrastructure: Buildings, facilities, vehicles, and large-scale physical systems
- Intellectual property: Patents, trademarks, copyrights, and proprietary business knowledge
How to use AI and machine learning in asset management
Artificial intelligence and machine learning shape how businesses manage their assets. While AI refers to a broader set of systems that mimic human intelligence, machine learning is the engine behind many of those systems. It trains algorithms on historic asset data so they improve continuously over time without requiring any reprogramming assistance from your tech teams.
Together, AI and machine learning both support teams in working faster and more precisely. Here are some of the ways enterprise teams use them in asset management today:
- Natural language processing (NLP): Analyze financial filings, legal documents, or maintenance logs to extract key information that supports asset tracking and compliance.
- Big data analysis: Process large and complex datasets across asset classes to uncover patterns, trends, or hidden risks.
- Idea generation: Identify new investment opportunities or asset strategies by pinpointing signals humans might miss in noisy datasets.
- Proxy signal identification: Use indirect indicators, like energy consumption or traffic patterns, to inform decisions when direct data is unavailable.
- Sentiment analysis: Interpret tone and intent from earnings calls, news, or analyst commentary to guide financial asset decisions.
- Knowledge graph construction: Connect related data across assets, vendors, and facilities to reveal dependencies and systemic risks.
- Reinforcement learning: Improve asset decision-making through iterative feedback loops that refine models over time.
The business-critical benefits of using AI in asset management
Artificial intelligence exists for a reason. It makes our lives easier by ridding us of the laborious, manual workflows that eat into our time and produce disappointing results. Here’s where the impact of AI is most visible for asset management teams.
Continuous monitoring
When asset information is only updated once a week, teams lose the chance to act while it still matters. AI changes that by processing asset signals as they arise instead of waiting for someone to spot the problem manually. That might mean triggering a maintenance alert from sensor data or flagging a shift in portfolio performance based on real-time market changes.
Greater efficiency
According to Mercer, 93% of managers expect AI to increase productivity across their asset operations — and for good reason. The technology frees up time for analysis and action instead of repetitive tasks like copying data between systems, cleaning up errors, or reconciling conflicting entries. AI handles all these tasks and many more in the background, so your teams can execute value-adding work instead.
Improved scalability
As asset portfolios grow, the complexity of managing them multiplies. Data comes from more sources, systems don’t always talk to each other, and governance starts to slip. It’s a headache that AI can relieve with a more consistent approach. The technology applies the same logic to thousands of records at once — tracking usage, status, and risk across locations without adding layers of manual review. Additionally, teams can onboard assets, expand into new regions, or integrate systems without rebuilding their internal processes every single time.
Predictive resilience
One of the biggest questions asset managers face is: how will our assets be impacted by what’s coming up? To find out, AI analyzes broader patterns related to shifting demand, regulatory changes, or external supply issues and identifies if and when these conditions will impact your assets. These early, actionable insights allow teams to adjust plans or rework budgets long before the disruption kicks in.

What are the challenges of AI in asset management?
AI excels at finding signals in the noise. But asset management teams can run into some problems when they try to jam AI into their workflows without laying the groundwork first. Here’s what to watch out for.
Data quality and availability
AI is only as good as the data it works with, which is problematic if your asset data is incomplete, outdated, or formatted inconsistently across platforms. 68% of asset managers agree that data quality and availability are among the biggest roadblocks to meaningful AI adoption. This holds true whether you’re tracking market movements, equipment usage, or lease agreements. If the data doesn’t arrive in the right shape, models lose their value quickly.
Compatibility issues
Even with solid data, most asset environments still rely on a patchwork of legacy systems and custom databases that make it harder for AI to connect the dots and do anything useful with the information. For this reason, 54% of asset managers say integration and compatibility are a significant barrier to AI implementation in their companies, according to Mercer.
Security and privacy risks
AI tools often need access to sensitive data, including contract details and vendor relationships. Mercer’s data reveals that protecting this information is a serious concern for 47% of asset managers, especially in organizations handling large volumes of regulated information.
Lack of explainability
47% of managers also struggle with justifying AI output to other key stakeholders. Asset decisions often need to be audited, defended, or explained, especially in regulated industries or when high-value assets are involved. But when AI operates like a black box, it’s harder to convey why you’ve made certain asset decisions or even confirm if they’re the best choice.
Difficulties sourcing skilled talent
Even the best AI tools are limited by the people using them. Asset managers face a growing skills gap in terms of understanding and applying AI in their daily workflows. According to PwC, 30% of asset managers admit they don’t have the expertise they need to adopt AI and execute their strategy plans.
6 examples of AI applications in asset management
AI can work its magic across multiple types of assets. Whether you’re managing portfolios, property, or physical infrastructure, AI supports organizations in making smarter decisions earlier and with less manual effort. Here’s what this innovative approach to asset management looks like across a variety of use cases.
1. Portfolio management
Market trends move faster than most teams can react. AI supports your portfolio management by scanning for subtle signals across price movements, earnings reports, macro indicators, and sentiment data. It also flags when a portfolio drifts from its targets, suggests rebalancing actions, and identifies high-potential trades others might miss. The result is crystal-clear judgment with all the noise stripped out.
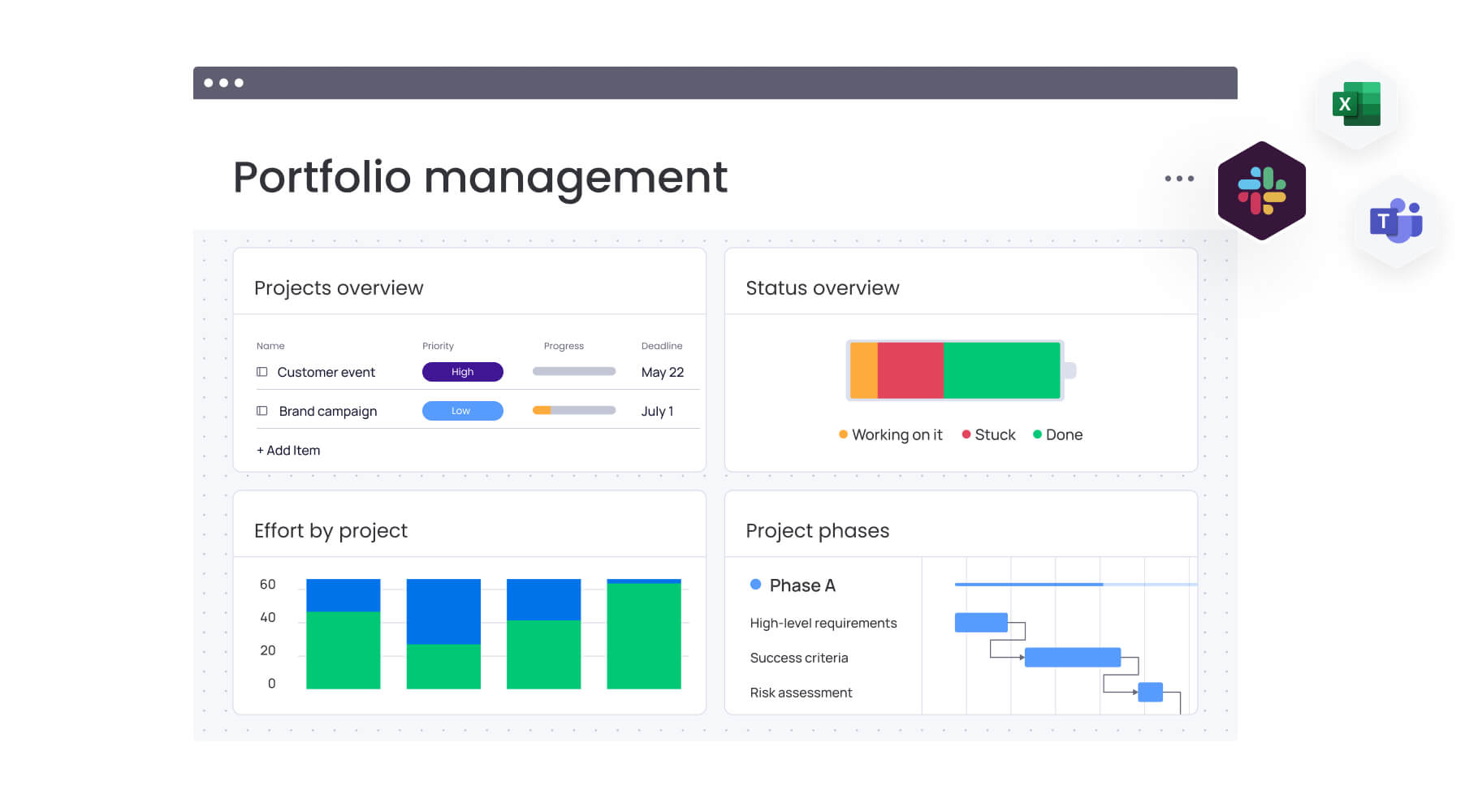
2. Risk management
Assets rarely fail without warning. AI gives asset managers an edge by spotting risk patterns before they become problems. It can run scenario models, stress test positions, and monitor for volatility across asset classes, all without waiting for manual intervention.
3. Capital project planning and asset investment strategy
Big decisions around capital assets rely on forecasts, but static plans fall apart when prices shift or delays hit. AI strengthens that process by simulating different scenarios in real time, helping teams understand the downstream impact of every change. Whether reworking budgets or reshuffling business priorities, leaders can adapt based on facts, not assumptions.
4. Digital asset monitoring
Digital assets, like software licenses, datasets, and subscriptions, are hard to track across multiple teams and systems.
AI brings that information into clear focus to keep everything streamlined and avoid any unnecessary spend. For example, the technology can monitor contract terms, flag potential violations, and highlight when assets are underused, overused, or at risk of compliance breaches.
5. Operational asset tracking
Manual asset logs are hard to maintain, especially in global enterprise companies. AI gives teams a running view of what’s being used, what’s idle, and what’s likely to need attention.
With performance data and location tracking in one place, operations teams can make faster calls about servicing, replacements, or reassignments without relying on outdated reports or guesswork.
6. Real estate and facilities
Buildings are assets, too, and they come with costs that add up fast when space is underused or maintenance is reactive. AI tracks how the company uses its facilities and when they need attention. It spots usage trends, identifies energy waste, and flags maintenance needs before they affect operations or budgets.
monday service: A smarter solution for AI in enterprise asset management
Effective asset management requires agility, visibility, and precision. monday service provides all three by bringing intelligent, AI-driven capabilities into your daily workflows, and helping teams streamline asset management at scale. Here are the specific benefits you’ll experience when you adopt our service management platform.
Gain instant visibility into your assets
monday service’s customizable dashboards present all the asset insights you need — in one central location. You’ll configure your perfect setup from 27+ different board views, 36+ columns, and 25+ widgets and view live metrics on everything from equipment uptime to upcoming maintenance needs. It’s the best way to spot underperforming or idle assets and align every asset decision with your operational efficiency and financial performance goals.
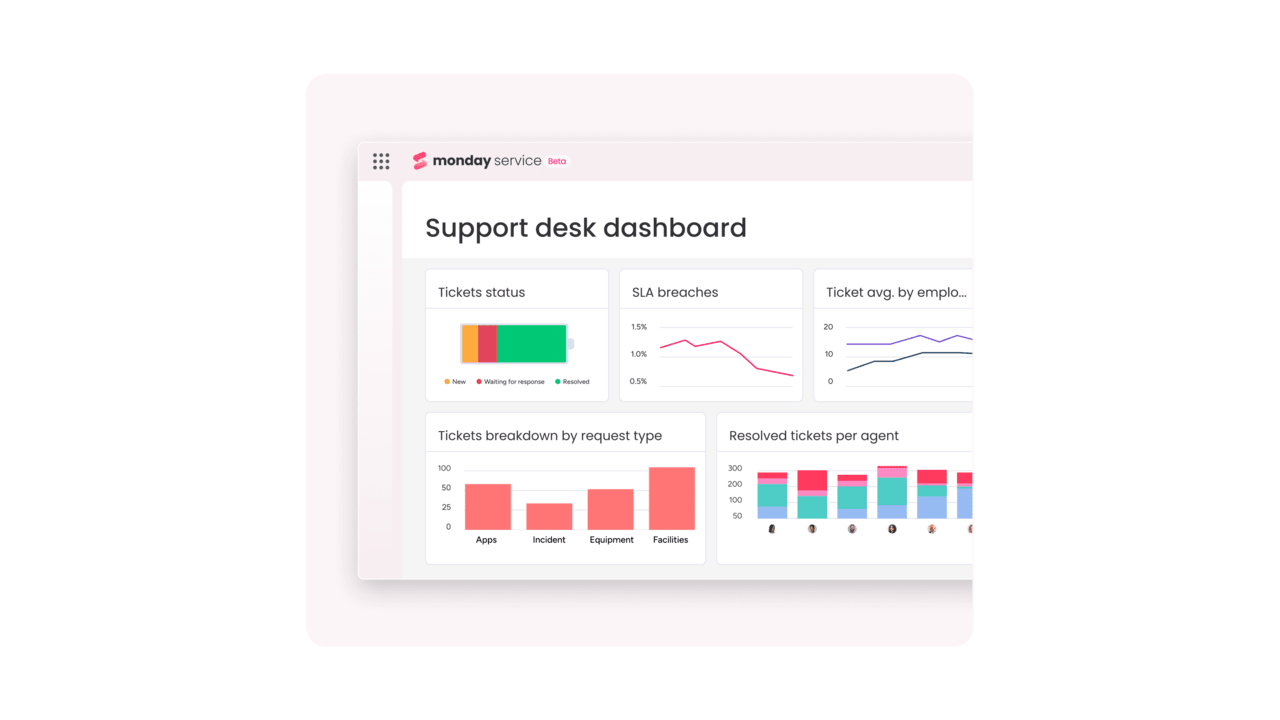
Eliminate asset resolution bottlenecks
Whenever you have an asset management issue to resolve, your tasks will always reach the right hands with monday service. Our AI-powered ticketing feature uses smart routing for each asset task — each is automatically classified, assigned, and escalated as needed, leading to faster, more effective resolutions.
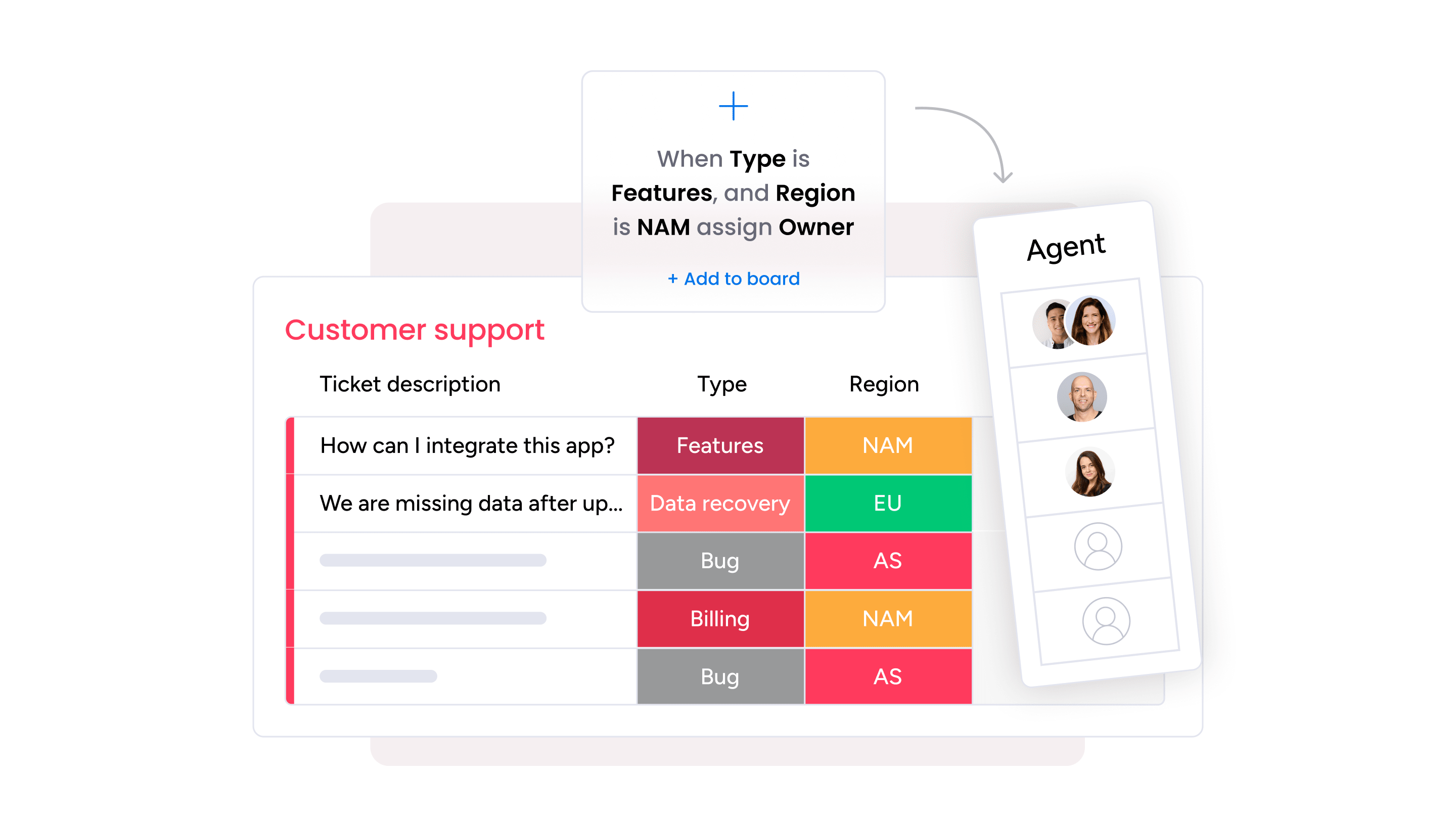
Stay ahead of risks with predictive asset monitoring
Instead of waiting for breakdowns or financial risks to materialize, anticipate them before they occur. monday service’s predictive analytics flag enterprise risks and performance anomalies early, so you can avoid them or find a solution.
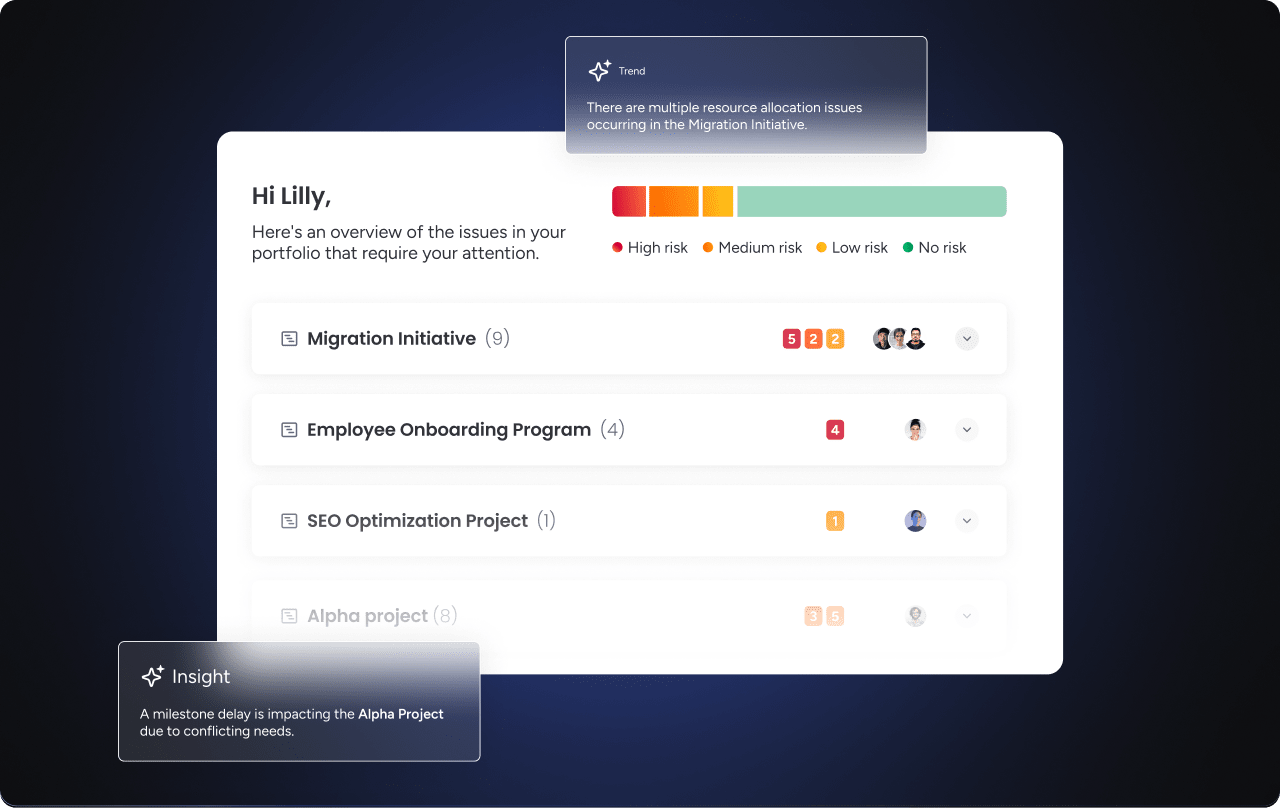
Boost productivity by automating asset management tasks
Instead of manually tracking your assets, create simple automations to keep tabs on your investments. For example, if a software license is close to its expiration date, you can use automation to create and assign a renewal task to the IT manager. This step removes the burden of constantly checking dates and sending out reminders.
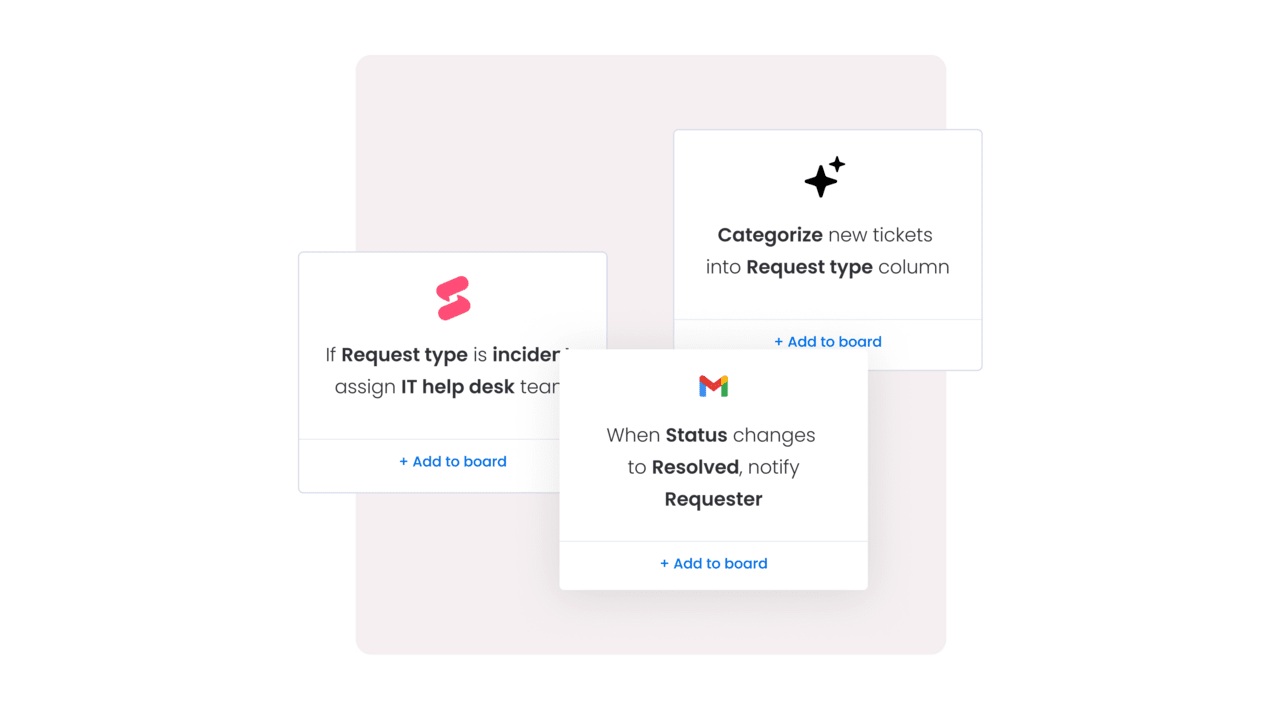
Simplify complex processes through seamless integrations
Disconnected data silos slow decisions down. But with seamless integrations into 72+ different systems like CRMs, ERPs, finance systems, and more, monday service connects and stores all your asset data, delivering a clear, unified view across your entire organization to support your decision-making processes.
AI helps asset managers spend less time tracking details manually. monday service puts these AI tools directly into your daily workflow, so managing your assets becomes simple and powerful. Get a free trial of monday service to take control of your assets today.
Try monday serviceFAQs
What are the benefits of AI in digital asset management?
AI makes digital asset management smarter. It automates tedious work, such as organizing, tagging, and tracking assets, so teams can focus on what really matters. The result is less time hunting for files and more time executing your strategy.
How will GenAI change asset management?
Generative AI allows asset managers to make decisions faster and more efficiently. It automates tasks like data analysis, portfolio adjustments, and risk assessments, all while providing more accurate and actionable insights. As the technology evolves, teams can focus on strategy and high-level decisions rather than getting bogged down in manual processes.
Will AI take over human expertise in asset management?
No, AI supports rather than replaces human expertise in asset management. While AI excels at analyzing large data sets and identifying trends, human judgment remains essential for interpreting complex situations and managing relationships.
What is the future of AI in wealth management?
The future of AI in wealth management lies in its ability to offer personalized financial advice and optimize portfolios in real-time. As AI becomes bigger and better, wealth managers will use it to automate routine tasks, predict market conditions, and provide tailored recommendations for clients.